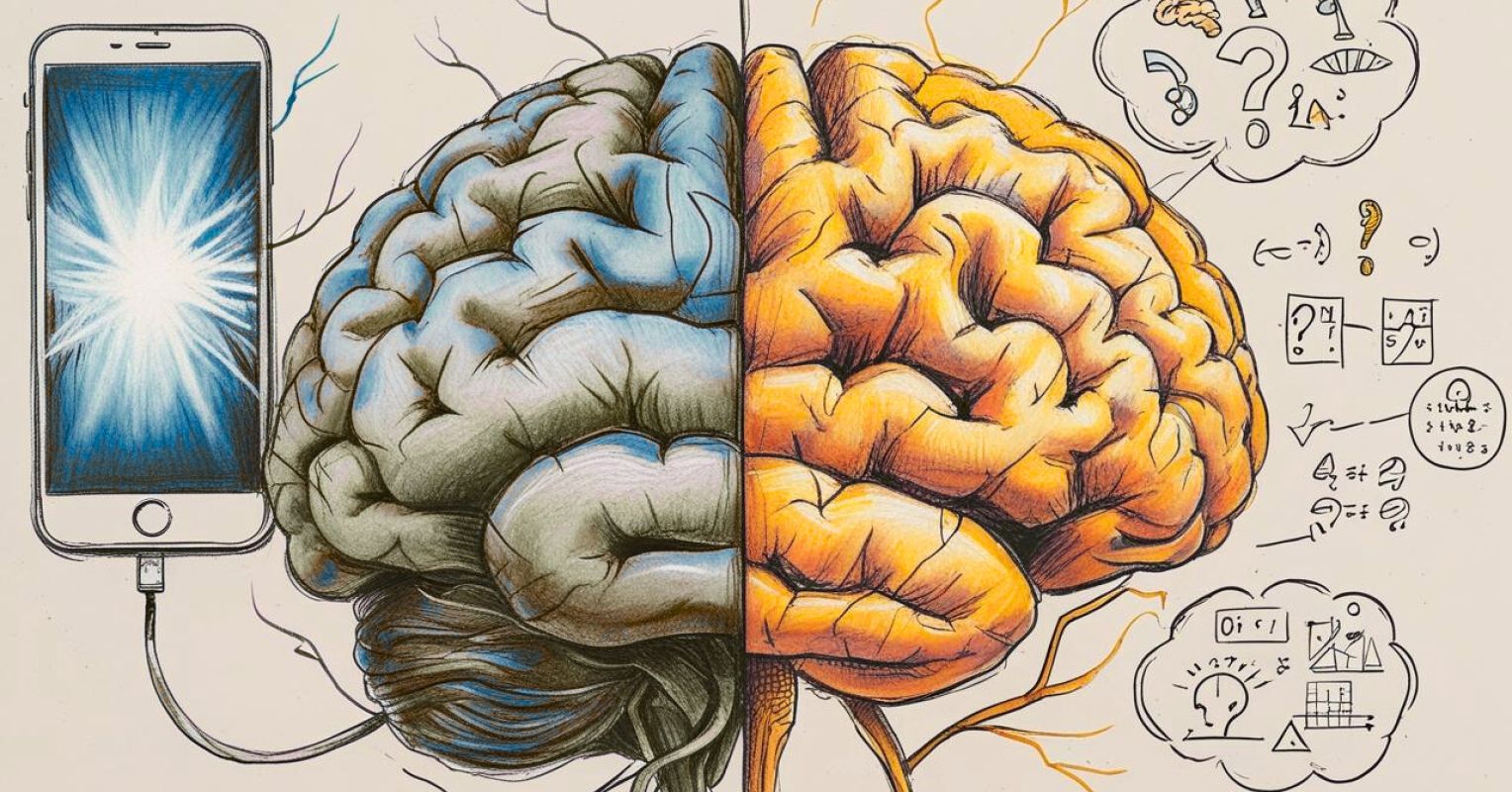
The Silent Atrophy of Critical Thinking
Emma, a college sophomore, stares at her screen. Her professor just assigned an essay on Kafka’s Metamorphosis and her fingers hover immediately over ChatGPT. ‘Why struggle,’ she thinks, ‘when AI can analyze it for me?’ This split-second decision mirrors a global cognitive shift:
We’re trading mental effort for convenience, and our brains are adapting in alarming ways.
What happens to a muscle when it’s not used? It weakens. It atrophies. It only recovers when its used again. So, what happens to a mind when thinking is outsourced? As you glance at ChatGPT, perhaps to write your next email or summarize your next work task, consider what cognitive muscles you might be allowing to weaken?
In a laboratory at Switzerland, participants stare at screens, making split-second decisions about whether to solve problems themselves or delegate them to artificial intelligence. Convenience is silently reshaping our intellectual architecture.
The Cognitive Cost of Convenience
“Cognitive offloading emerged as a mediating factor, particularly among younger participants who exhibited lower critical thinking skills due to habitual reliance on AI,” writes Gerlich in his 2025 Swiss study examining a stratified sample of 666 participants across age groups.
His research reveals a significant negative correlation between frequent AI tool usage and critical thinking abilities. This was measured through validated instruments like the Halpern Critical Thinking Assessment.
These changes represents the evolution of what Sparrow and colleagues first identified in 2011 as the “Google effect”—our tendency to forget information we know is retrievable online. But Gerlich’s findings suggest something more concerning. The ‘Google effect’ extends to critical thinking, where individuals may prioritize knowing where to find information over understanding or analyzing it deeply.
The smartphone in your pocket has become an extension of your cognitive system. Google Search required us to sift through results, evaluate sources, and synthesize information. This exercised our cognitive muscles of analysis and evaluation. But today’s LLMs perform these intellectual tasks for us, delivering pre-packaged insights without asking for our mental participation at all.
The transition from search engines to generative AI demonstrates a shift from tools that required collaborative thinking to technologies that encourage passive consumption of machine-generated thought. This subtle but significant difference will change us from active participants in knowledge creation to mere recipients of machine output. What affects will this have on our neural pathways responsible for critical thinking, evaluation, and synthesis?
What started as outsourced memory has evolved into outsourced reasoning.
Your Brain Loves (and Fears) Cognitive Offloading
Why do we so readily surrender our cognitive autonomy? Perhaps because delegation feels like empowerment. Each time AI completes a task we once performed manually, we experience a momentary efficiency gain. The design of LLMs induce a dopamine-fueled reward that reinforces our dependence, similar to gamification.
Wahn et al. (2023) experimentally demonstrated that humans willingly offload attention-demanding tasks to algorithms when cognitively overloaded. In their study, participants performing a multiple-object tracking task offloaded some but not all targets to an AI partner, improving their individual tracking accuracy by 18% despite wide ‘aversion’ to algorithmic assistance. This suggests that cognitive load overrides initial reluctance to delegate when there is a perceived convenience to doing so. The authors conclude that “task load is a critical factor in offloading behavior” with implications for AI-assisted workflows in high-stakes fields like education and healthcare.
Beneath this convenience hides genuine anxiety. Gerlich’s research uncovered substantial public concerns about AI that extend beyond technical risks to deeper psychological worries. In both studies, participants often downplayed these anxieties in public settings but expressed significant concerns anonymously. Gerlich suggested our society hasn’t yet developed the vocabulary to articulate our anxiety with these technological changes.
Perhaps this anxiety is just a reflection of a recognized truth: AI tools are changing us.
Reclaiming Our Cognitive Future
What distinguishes Gerlich’s work is its rejection of technological determinism. Rather than positioning AI as an unstoppable force reshaping cognition, his research highlights our agency in determining outcomes through intentional design and policy.
The path forward requires neither the embrace nor rejection of AI. Instead, we need thoughtful integration of these tools that preserves human cognitive autonomy. This means designing educational experiences that teach students not just how to use AI but when not to use it. It means that educational environments value human judgment alongside algorithmic analysis.
Four Strategies for AI-Resistant Thinking
How can we harness AI’s efficiency while preserving the cognitive independence that drives creativity and innovation? The answer lies within human-centered learning. Recent research on integrating AI in education suggests four actionable approaches to balance AI efficiency with cognitive independence:
- Implement “AI-free zones” for deep thinking: Designate specific classroom activities and assessments where AI tools are intentionally absent. Research shows that deliberate practice without technological assistance strengthens neural pathways responsible for critical analysis (Bhuman, 2024). These zones create essential opportunities for students to develop independent thinking without algorithmic shortcuts.
- Teach comparative judgment between AI and human outputs: Design exercises where students evaluate both AI-generated and human-created analyses of the same material. This helps students identify the qualitative differences in reasoning processes and develop metacognitive awareness of when human judgment adds distinctive value beyond algorithmic processing.
- Develop “AI-proof” assessments focusing on process over product: Shift evaluation metrics to emphasize students’ ability to document their thinking journey, explain reasoning, and justify conclusions. Assessment designs should value the “why” behind answers rather than just output or correctness.
- Foster collaborative human problem-solving communities: Create structured opportunities for student teams to tackle complex problems through dialogue, debate, and iterative refinement with each other and with AI. have developed the Dialogic Learning Prompts for this purpose.
As our devices become more capable, the most valuable human cognitive skills may shift from information processing (knowing what) to meaning-making (knowing why). This represents an evolution in what we consider essential human intelligence in the algorithmic age.
When you next reach for AI to complete a cognitive task, pause to consider: What capacities are you developing, and which might you be surrendering? Share your answer and your strategies below.