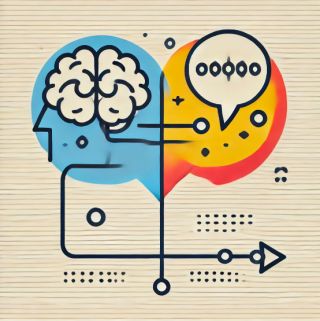
Effective learning isn’t just about finding the easiest path—it’s about the right kind of challenge. Two prominent theories—Desirable Difficulties (DDF) and Cognitive Load Theory (CLT)—offer valuable frameworks for understanding how we learn and retain information. A recent paper has highlighted the importance of managing “difficulty” in learning environments, revealing that an appropriate balance of challenge and support can foster deeper cognitive engagement and long-term memory retention.
The importance of these two academic theories got me thinking. The critical interplay between difficulty and cognitive load seems ripe to explore in the context of Large Language Models (LLMs) and how they can serve a complementary tool in optimizing learning.
Desirable Difficulties and a Framework for Learning
The Desirable Difficulties framework, introduced by psychologist Robert Bjork, presents that learning is most effective when students encounter moderate challenges that require active engagement. This idea challenges the traditional view that learning should be as easy as possible. Instead, Bjork argues that learning becomes deeper and more enduring when it involves some level of difficulty, such as trying to recall information from memory, spacing study sessions, or testing oneself. The key is that the difficulty should be manageable—neither too overwhelming nor trivial.
Research suggests that these “desirable difficulties” help us process information more effectively, helping learners form stronger memories. The tasks that force learners to work harder, even though they may appear more difficult, often lead to better long-term retention. The Retrieval Effort Hypothesis (REH) expands on this idea by arguing that the effortful retrieval of information strengthens memory networks, making them more accessible in the future.
The crucial element here is that the difficulty involved in learning should be intentional, strategic, and designed to push learners beyond their comfort zones. When tasks become too easy, learners fail to engage deeply with the material. When they become too hard, learners risk cognitive overload and frustration. Therefore, the sweet spot lies in moderate difficulty—just enough to stimulate cognitive effort without overwhelming the learner.
Cognitive Load Theory and Managing “Thinking” Resources
Cognitive Load Theory (CLT), developed by John Sweller, offers a complementary perspective, emphasizing the importance of managing cognitive resources to optimize learning. CLT argues that the brain has a limited capacity for processing information, and when cognitive load exceeds this capacity, learning is hindered. The theory divides cognitive load into three types:
- Intrinsic Load: The inherent difficulty of the material itself. More complex topics generally have higher intrinsic load.
- Extraneous Load: The load created by the way information is presented, such as poorly designed instructional materials or unnecessary distractions.
- Germane Load: The cognitive effort dedicated to processing information, organizing it, and making sense of it. This type of load contributes directly to learning.
According to CLT, effective instructional design should minimize extraneous load and focus on germane load, directing cognitive resources toward understanding the material and forming cognitive schemas. The intrinsic load is unavoidable but should be managed by breaking complex topics into smaller, more manageable segments.
Integrating LLMs into Learning
While both Desirable Difficulties and Cognitive Load Theory offer valuable insights into how difficulty affects learning, their true potential can be realized when combined with modern educational tools—specifically, Large Language Models.
LLMs provide a powerful mechanism for implementing both desirable difficulties and cognitive load management. These models are capable of offering personalized, dynamic interactions that adjust to the learner’s needs, fostering an ideal learning environment that balances challenge and support.
Enhancing Desirable Difficulties—LLMs excel at introducing controlled difficulty into the learning process. For instance, rather than providing simple answers, LLMs can ask follow-up questions, prompt users to elaborate on their responses, or offer alternative perspectives. This interaction not only deepens engagement but also mirrors the principles of desirable difficulties by requiring learners to think critically and reflect on their understanding. An LLM can also provide spaced repetition, allowing learners to revisit concepts at increasing intervals, which further enhances retention.
In this way, LLMs can act as cognitive partners, guiding learners through a process of active problem-solving and intellectual exploration. The challenge is neither too easy (which leads to boredom and disengagement) nor too difficult (which results in frustration and overload)—but just right, encouraging growth while maintaining motivation.
Managing Cognitive Load—LLMs are also uniquely equipped to manage cognitive load. They can offer tailored responses that adjust the complexity of the material based on the learner’s proficiency and needs. For example, if a learner is struggling with a particular concept, the LLM can break it down into smaller, more digestible parts, lowering the intrinsic load. If the learner is progressing well, the LLM can introduce more complex scenarios, increasing the challenge while keeping the learner engaged.
Additionally, LLMs can help reduce extraneous load by providing well-structured, contextually relevant explanations and removing distractions. The clarity and organization of LLM responses ensure that learners can focus on the most important concepts without becoming overwhelmed by irrelevant details or confusing language.
Fostering Germane Load—By guiding learners through thoughtful interactions, LLMs can promote germane load, or mental effort, directing cognitive resources toward deeper processing and understanding. When LLMs ask thought-provoking questions or provide nuanced explanations, they encourage learners to think more critically, make connections, and organize their knowledge. This type of engagement is essential for fostering meaningful learning, as it encourages the learner to invest cognitive effort into understanding the material and integrating it into existing knowledge frameworks.
LLMs Making Learning “Just Right” for Learning
The theories of Desirable Difficulties and Cognitive Load offer valuable insights into the nature of learning, emphasizing the importance of manageable challenges and effective cognitive resource management. By integrating Large Language Models into the learning process, we may create an ideal environment that both challenges learners and optimizes their cognitive resources. The result is a learner-centric, adaptive learning experience. It’s this powerful dynamic that encourages deep engagement, long-term retention, and critical thinking that I’ve referred to as the “Goldilocks Zone of Learning“—where a unique balance is supported and even driven by AI.
It’s my thesis that LLMs are “just right” in their ability to strike the balance between difficulty and support. And this positions them as an invaluable tools for the modern, life-long learners of today.